Ever since AI has found its way into the corporate world, automation projects have been in the hands of data scientists. These new entrants in the business world, although bringing sharp expertise in intelligent data systems, don’t necessarily provide the same judgment as experienced business people.
This is especially critical when it comes to identifying output or results that don’t make sense to a market insider. Fortunately, solutions have been designed to make the benefits of AI accessible to any worker.
These no-code platforms provide visual and drag-and-drop interfaces, which allow users to intuitively program, label, and train learning models.
Here’s how they can spread AI use cases in companies.
Auto-ML vs. No Code AI
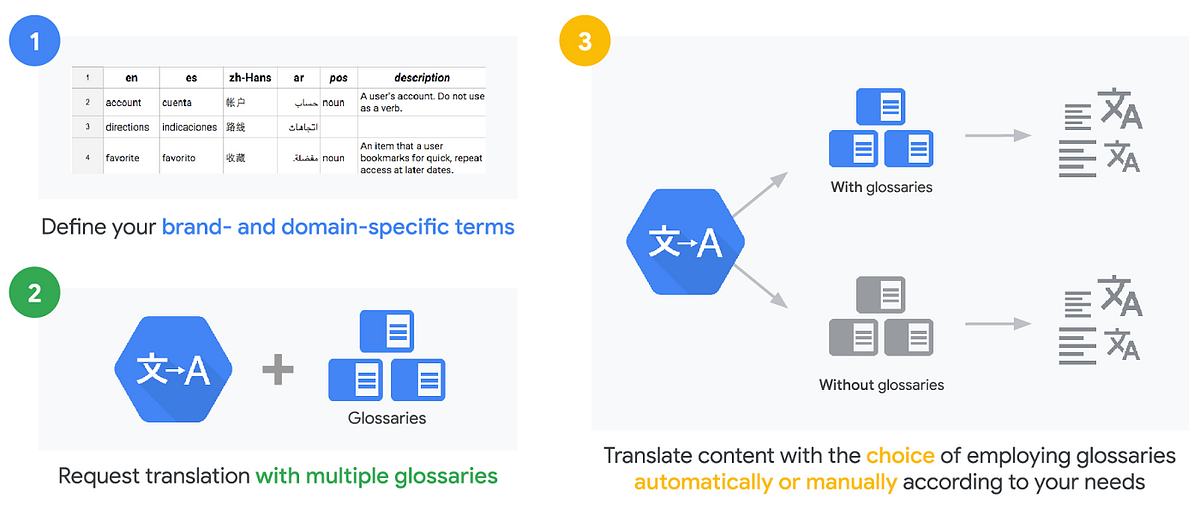
If there is one truth widely accepted in data science, it’s that training a machine learning model takes a lot of time and resources.
Whether it’s writing the code, cleaning the data, creating the labels, or updating the models, data scientists often spend their time on fairly repetitive tasks. Quite paradoxical for people who want to automate and streamline processes…
Automated Machine Learning technologies were invented by AI giants (Google, Microsoft, or IBM) to save time for their data experts. They provide solutions that automatically train learning models for narrow and specialized tasks. In particular, they rely on a library of existing algorithms to select the most suitable model, generate labeled data, and thus improve the model through a step-by-step refinement.
However, the specificity of AutoML platforms is that they are intended for data science specialists. To manage their use and optimize their results, users must know how to master all the technical tasks of machine learning (data cleaning, labeling, and debugging), and assess uncertainty margin and bias.
This is where the difference with no code AI lies: no code AI makes a neural model building accessible to anyone in the enterprise. With a visual, plug-and-play, and modular interface, users have everything in their hands to implement a machine-learning application in their workflow.
These No Code AI solutions, therefore, promise decisive benefits for companies lacking talent or resources.
The Business Benefits of No Code
Recruiting and maintaining a data scientist is a long and expensive project. This is especially true for mid-sized companies that might face talent retention issues and a fund shortage.
Yet, the deployment of AI can generate huge opportunities for these companies. The specificity of machine learning is to generate process improvements in all business aspects. The use cases are thus numerous:
- Automating contact scraping and qualification to maximize the sales team’s prospection
- Real-time advertising budget optimization
- Managing repetitive administrative operations such as claims or invoice analysis with the least human intervention
- Analyzing and monitoring at scale customer reviews and complaints
- Categorizing extensive product catalogs
The ambition of these no-code AI platforms is to provide the benefits of automation by starting from small but impactful applications. With a visual drag and drop interface, workers usually only need a few days of work to see the ROI of each automation project. This way, companies avoid investing a lot of resources on projects with no payoff and get the maximum potential out of applications that are worth the money.
Data Models and Bias
Despite a strong value proposition, leveraging the opportunities of no-code AI in your company can require some caution. While data scientists are aware of the potential biases that algorithm models convey and can prevent them, that’s not the case for the average user.
As No Code AI tools automate the programming of models, someone still needs to refine the data and debug the models. For visual recognition algorithms alone, the output quality depends a lot on the data set quality. Clear images without too much variation, clear annotations without noise, are as important to ensure the accuracy of the model. And this is without adding the risk of bias added by human selection.
For example, take the case of assessing a candidate’s profile from a picture included in their resume. This photo can impact the entire value of the resume as the model incorporate in its judgment the annotator’s implicit assumptions.
For this reason, No-code AI users o need to constantly reevaluate the relevance of their model’s results. They need to stay humble and question the results when their model seems to perform too well to be true.
One thing to know is that the accuracy of machine-learning models depends on the nature of the problem to be solved. So you need to refine the conditions of your problem to arrive at a result that best meets the need while being realistic.
Examples of No Code AI platforms
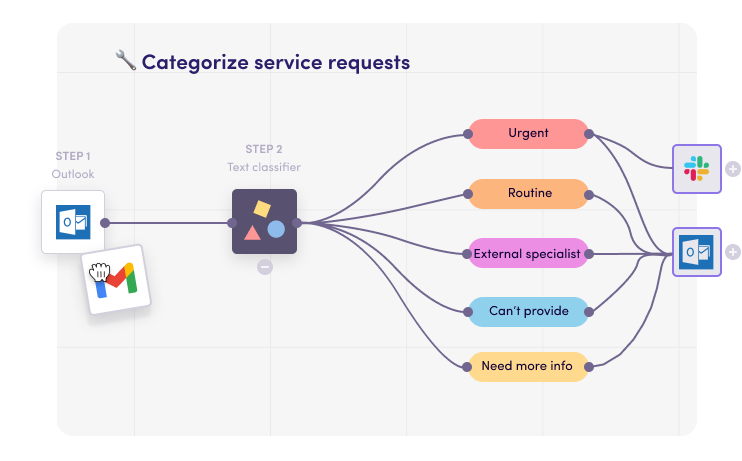
There are already numerous No Code AI solutions on the market, and each of them brings unique features.
Solutions like Obviously AI or Akkio add the predictive powers of AI to your common spreadsheets. You simply need to connect your data resources to the app and it will build a predictive model from them. You can then figure out the probability of customer churn, define optimum price balances, or spot employees at higher risk of turnover.
Tools like Lobe or Nanonets rely on the power of computers to make predictions on unstructured data. With a user-friendly and accessible interface, Lobe allows you to recognize or classify objects from images or videos. Nanonets does the same thing but manage unstructured documents.
Other platforms such as Levity, Clarifai, or Google Cloud ML make all these data science capabilities available on a single dashboard. Users can perform both visual quality inspection, facial recognition, content moderation, or predictive maintenance across images, video, audio, and textual documents. They are the ultimate tools to leverage the big data owned by medium-sized companies.
What are the consequences of these tools with unlimited possibilities for the future of work? Workers have in their hands all the intelligence of the data and can maximize their input. They can make the most out of data-driven automation.